Data Fidelity as an Enabler: Transforming Demand Forecasting and Risk Mitigation
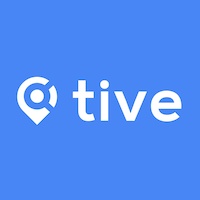
April 16, 2025
July 8, 2025
x min read
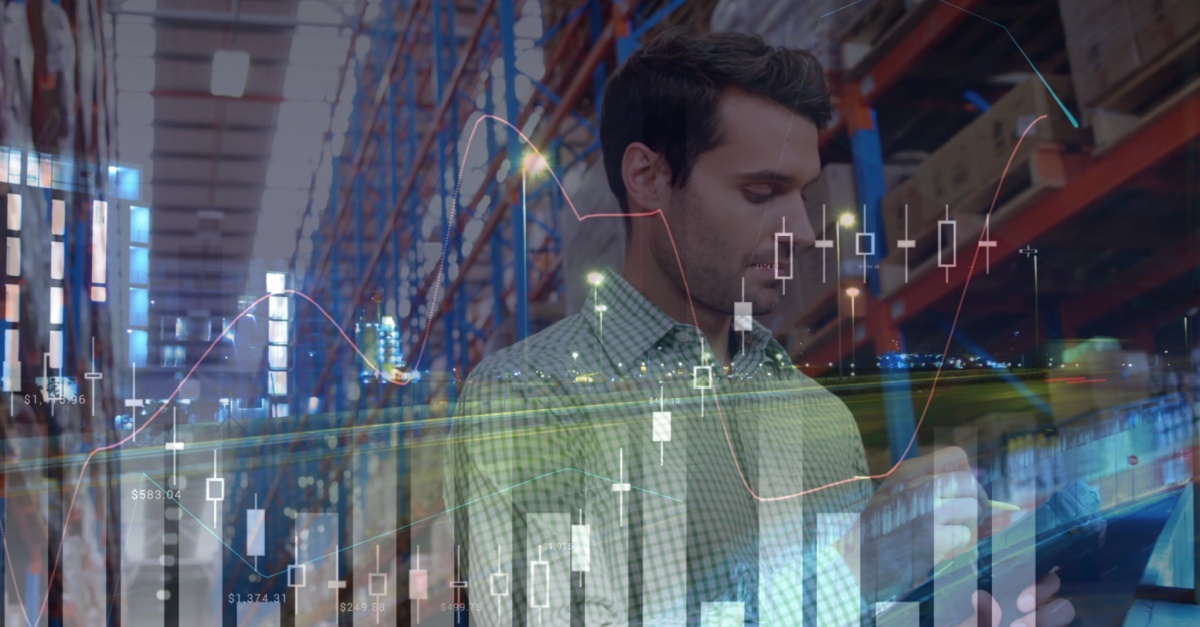
It’s Sunday night, you’re starving, and the only “food” in your fridge is leftover Indian from over a week ago. But you’re desperate and don’t have the patience for DoorDash, so you reheat it anyway—and immediately regret that move.
That’s exactly what you’re doing to your expensive AI system by feeding it junky data.
Think about it: you wouldn’t expect Gordon Ramsay to create a masterpiece from questionable leftovers, so why expect your AI to work miracles with incomplete or outdated information?
Even though AI adoption has jumped from 35% to 45% in logistics, there’s a big difference between transformative AI and trash—and it all lies in data fidelity and integrity.
Throw all the money you want at fancy algorithms. We dare you. You’ll quickly find out the hard way that no amount of algorithmic sophistication can turn digital junk food into filet mignon.
You can only expect AI to give you optimized inventory, accurate forecasts, and flawless responses to disruptions by taking a data fidelity-first approach.
How Quality Data Fuels ML Success Stories That’ll Make Your Supply Chain Jealous
Before you throw another bag of cash at fancy AI solutions, consider what the early ML adopters already know: quality data means everything. When companies pair pristine data—from sales histories to real-time tracking—with machine learning, magic happens. You may even find your logistics costs decrease by 15%, inventory levels reduce by 35%, and service levels improve by 65%.
No More Empty Shelves or Dusty Overstock: Smart Inventory That Works
You’ve likely played Goldilocks with your inventory levels: too much ties up capital and too little costs sales. ML algorithms solve this puzzle by crunching historical sales, seasonal patterns, and real-time data to calculate precise reorder points and safety stock levels—but only when fed accurate, comprehensive data.
That’s the payoff of high-fidelity data for you—predictions you can actually trust.
Alibaba nailed this approach, using machine learning to analyze customer behavior and predict demand with uncanny accuracy. Meanwhile, UK grocery chain Ocado deployed ML in automated warehouses to balance stock levels, leading to faster order fulfillment and reduced waste. The secret weapon? AI-driven inventory tools that evaluate demand forecasts, lead times, and safety stock requirements, then continuously adjust recommendations in real time.
Psychic Not Required: Forecasting That Hits the Mark
Want forecasts that don’t make you laugh six months later? ML-powered demand prediction reaches up to 92.5% accuracy when given the right ingredients. The recipe calls for rich, varied data: internal sales histories and POS data mixed with external factors like market trends, social sentiment, weather patterns, and real-time shipment tracking.
UPS cracked this code by analyzing traffic patterns, weather, and delivery windows for route optimization—all of which feeds back into smarter demand projections. Jaguar Land Rover took a similar path, using AI to predict demand for specific car models, and aligning production with market trends to avoid costly overproduction.
The results speak volumes: advanced forecasting frameworks like Long Short-Term Memory (LSTM) can cut inventory holding costs by 18%—while boosting order fulfillment rates by 22%.
Disaster? What Disaster? Disruption-Proof Supply Chains
Supply chain disruptions used to send everyone into panic mode. Now, ML-driven predictive analytics spot trouble before it spirals—whether it’s supplier shortfalls, weather disruptions, or cargo theft threats.
The key ingredient? Real-time visibility through IoT and telematics data gives ML models the “eyes” they need to detect problems in transit.
Chemical manufacturing supply chains have mastered this approach, analyzing supplier performance and geopolitical events to handle raw material shortages proactively. DHL’s Greenplan Algorithm uses ML for route optimization to sidestep weather- or traffic-related delays before they impact customers. Meanwhile, predictive maintenance powered by AI pinpoints equipment issues before they escalate—keeping deliveries on schedule.
There is No Magic AI Fairy: Five Steps to Better Data Today
You’ve seen what top performers accomplish with quality data fueling their ML systems. The gap between leaders and laggards grows wider every day—but you don’t need to fall behind. No magic AI fairy can give you a leg up. Yet, you can follow a straightforward data fidelity road map to join the success stories we mentioned without waiting for a miracle.
- Clean your digital junk drawer: Audit your current data sources, quality, and compatibility. Most companies sit on gold mines of information buried under piles of duplicate, outdated, or contradictory data. A thorough cleaning creates the foundation that makes every ML initiative possible.
- Put eyes everywhere: Deploy IoT sensors that feed continuous, real-time data to your systems. Companies report significant reductions in inventory costs when they can see what’s happening across their supply chains—instead of relying on weekly reports and educated guesses.
- Pick one problem: Start with a focused pilot progrm tackling inventory optimization or demand forecasting. Companies that begin with targeted projects show much higher success rates than those attempting supply chain-wide transformations.
- Upgrade your forecasting: Add predictive analytics tools for supply chain challenges. The right tools cut logistics costs through capabilities such as route optimization and maintenance scheduling that prevent problems—rather than reacting to them.
- Turn data nerds into leaders: Create a culture in which accurate data collection gets celebrated—not ignored. When frontline teams understand how their input impacts ML performance, data quality improves without spending money.
The Burning Question: Premium AI or Premium Data? (Spoiler: It’s Both)
Data fidelity makes or breaks your AI logistics dreams. That’s a clear-cut fact. While everyone else throws money at shiny algorithms, the winners quietly obsess over what they feed those hungry systems to optimize their supply chains as much as possible. Without quality data, even the fanciest AI becomes nothing more than an expensive digital paperweight gathering dust—while your competitors sprint ahead.
At Tive, we’re fanatical about real-time shipment visibility—and collecting the highest-quality data that makes AI work its best. Our trackers don’t just tell you where your shipment is: they tell you where it is right now, what condition it’s in, and whether you need to worry about it. Our paper-thin temperature loggers mean you’ll never again hear, “We think it might have overheated somewhere between Chicago and Denver.” And our intuitive cloud platform turns overwhelming data streams into “oh, that makes perfect sense” insights your team can use immediately.
And whether you’re shipping lifesaving pharmaceuticals, perishables, or electronics that mysteriously “fall off trucks” (cargo theft, anyone?), our live monitoring team knows what to expect. We’ve seen your nightmares—and built solutions to stop them.
Ready to stop feeding your AI system digital leftovers? Let’s talk. Get started with Tive today.